Capturing human response to Winter Storm Frankie based on X (formerly known as Twitter) data
DOI:
https://doi.org/10.5055/jem.0827Keywords:
winter storm, Frankie, response, X, big dataAbstract
This study delves into how people responded to Winter Storm Frankie in the United States based on X (formerly known as Twitter®) data according to a multitude of regions, periods, sociodemographic characteristics, census regions, and geographical scales. This study finds that people actively respond to natural disasters on X during the winter storm week. Specifically, the highest number of keywords during the winter storm week is 1.6 times greater than the second-highest number of keywords during the prewinter storm week. Second, the spatial distribution of tweets exhibits significant fluctuations across different periods. For instance, in the prewinter storm week, more tweets are posted in the West region, while in the winter storm week, the Northeast region experiences a higher volume of uploads. Third, regional variables exert a substantial influence on the number of tweets. For instance, Ohio and Montana demonstrate higher elasticity than Pennsylvania. Fourth, many sociodemographic variables, such as gender, age, education, and income, are associated with individual responses. For example, a 1 percent increase in males corresponds to a 0.01 percent increase in tweets.
References
Reuters: Natural disasters cause $210 billion in damage in 2020, insurer says. 2021. Available at https://www.reuters.com/article/us-munich-re-disaster-idUSKBN29C17O. Accessed April 4, 2021.
Statista: Annual number of natural disaster events globally from 2000 to 2020. 2021. Available at https://www.statista.com/statistics/510959/number-of-natural-disasters-events-globally/. Accessed April 4, 2021.
Alexander DE: The l’Aquila earthquake of 6 April 2009 and Italian government policy on disaster response. J Nat Resour Policy Res. 2010; 2(4): 325-342. DOI: https://doi.org/10.1080/19390459.2010.511450
Burby RJ: Hurricane Katrina and the paradoxes of government disaster policy: Bringing about wise governmental decisions for hazardous areas. Ann Am Acad Polit Soc Sci. 2006; 604(1): 171-191. DOI: https://doi.org/10.1177/0002716205284676
Kusumasari B, Alam Q: Bridging the gaps: The role of local government capability and the management of a natural disaster in Bantul, Indonesia. Nat Hazards. 2012; 60(2): 761-779. DOI: https://doi.org/10.1007/s11069-011-0016-1
Brown P, Daigneault AJ, Tjernström E, et al.: Natural disasters, social protection, and risk perceptions. World Dev. 2018; 104: 310-325. DOI: https://doi.org/10.1016/j.worlddev.2017.12.002
Cameron L, Shah M: Risk-taking behavior in the wake of natural disasters. J Hum Resour. 2015; 50(2): 484-515. DOI: https://doi.org/10.3368/jhr.50.2.484
Ray B: Response of a resilient community to natural disasters: The Gorkha Earthquake in Nepal. Prof Geogr. 2017; 69(4): 644-654. DOI: https://doi.org/10.1080/00330124.2017.1298452
Spence PR, Lachlan KA, Lin X, et al.: Variability in X (formerly known as Twitter) content across the stages of a natural disaster: Implications for crisis communication. Commun Q. 2015; 63(2): 171-186. DOI: https://doi.org/10.1080/01463373.2015.1012219
Takahashi B, Tandoc Jr EC, Carmichael C: Communicating on X (formerly known as Twitter) during a disaster: An analysis of tweets during typhoon Haiyan in the Philippines. Comput Hum Behav. 2015; 50: 392-398. DOI: https://doi.org/10.1016/j.chb.2015.04.020
Zahra K, Imran M, Ostermann FO: Automatic identification of eyewitness messages on X (formerly known as Twitter) during disasters. Inform Process Manag. 2020; 57(1): 102107-102115. DOI: https://doi.org/10.1016/j.ipm.2019.102107
Gray CL, Mueller V: Natural disasters and population mobility in Bangladesh. Proc Natl Acad Sci USA. 2012; 109(16): 6000-6005. DOI: https://doi.org/10.1073/pnas.1115944109
Solís D, Thomas M, Letson D: An empirical evaluation of the determinants of household hurricane evacuation choice. J Dev Agric Econ. 2010; 2(5): 188-196.
United States Department of Homeland Security: Natural disasters. 2021. Available at https://www.dhs.gov/natural-disasters. Accessed April 4, 2021.
Reliefweb: Weather, climate & catastrophe insight. Annual Report. 2020. Available at https://reliefweb.int/report/world/weather-climate-catastrophe-insight-2020-annual-report. Accessed April 4, 2021.
National Oceanic and Atmospheric Administration: US billion-dollar weather and climate disasters in historical context. 2020. Available at https://www.climate.gov/news-features/blogs/beyond-data/2020-us-billion-dollar-weather-and-climate-disastershistorical#:~:text=The%20billion%2Ddollar%20disaster%20events,year%20average%20of%20%2445.7%20billion. Accessed April 4, 2021.
Crow DA, Albright EA: Intergovernmental relationships after disaster: State and local government learning during flood recovery in Colorado. J Environ Policy Plann. 2019; 21(3): 257-274. DOI: https://doi.org/10.1080/1523908X.2019.1623660
Deen S: Pakistan 2010 floods. Policy gaps in disaster preparedness and response. Int J Disaster Risk Reduct. 2015; 12: 341-349. DOI: https://doi.org/10.1016/j.ijdrr.2015.03.007
Rivera JD, Miller DS: A brief history of the evolution of United States’ natural disaster policy. J Public Manag Soc Policy. 2006; 12(1): 5-14.
United Nations Office for Disaster Risk Reduction: The Human Cost of Natural Disasters: A Global Perspective. Geneva: UNDRR, 2015.
Schultz J, Elliott JR: Natural disasters and local demographic change in the United States. Popul Environ. 2013; 34(3): 293-312. DOI: https://doi.org/10.1007/s11111-012-0171-7
Miao Q, Hou Y, Abrigo M: Measuring the financial shocks of natural disasters: A panel study of US states. Natl Tax J. 2018; 71(1): 11-44. DOI: https://doi.org/10.17310/ntj.2018.1.01
Tilcsik A, Marquis C: Punctuated generosity: How mega-events and natural disasters affect corporate philanthropy in US communities. Adm Sci Q. 2013; 58(1): 111-148. DOI: https://doi.org/10.1177/0001839213475800
Boustan LP, Kahn ME, Rhode PW, et al.: The effect of natural disasters on economic activity in US counties: A century of data. J Urban Econ. 2020; 118: 103257. DOI: https://doi.org/10.1016/j.jue.2020.103257
Weinkle J, Landsea C, Collins D, et al.: Normalized hurricane damage in the continental United States 1900–2017. Nat Sustain. 2018; 1(12): 808-813. DOI: https://doi.org/10.1038/s41893-018-0165-2
Ashktorab Z, Brown C, Nandi M, et al.: Tweedr: Mining X (formerly known as Twitter) to inform disaster response. In ISCRAM, University Park, Pennsylvania, USA, 2014: 269-272.
Doan S, Vo BKH, Collier N: An analysis of X (formerly known as Twitter) messages in the 2011 Tohoku Earthquake. In International Conference on Electronic Healthcare, Heidelberg, Berlin, Germany. 2011: 58-66. DOI: https://doi.org/10.1007/978-3-642-29262-0_8
Karami A, Shah V, Vaezi R, et al.: X (formerly known as Twitter) speaks: A case of national disaster situational awareness. J Inform Sci. 2020; 46(3): 313-324. DOI: https://doi.org/10.1177/0165551519828620
Murthy D, Longwell SA: X (formerly known as Twitter) and disasters: The uses of X (formerly known as Twitter) during the 2010 Pakistan floods. Inform Commun Soc. 2013; 16(6): 837-855. DOI: https://doi.org/10.1080/1369118X.2012.696123
Pourebrahim N, Sultana S, Edwards J, et al.: Understanding communication dynamics on X (formerly known as Twitter) during natural disasters: A case study of hurricane sandy. Int J Disaster Risk Reduct. 2019; 37: 101176. DOI: https://doi.org/10.1016/j.ijdrr.2019.101176
Kersten J, Klan F: What happens where during disasters? A workflow for the multifaceted characterization of crisis events based on X (formerly known as Twitter) data. J Contingencies Crisis Manag. 2020; 28(3): 262-280. DOI: https://doi.org/10.1111/1468-5973.12321
Alam F, Ofli F, Imran M: CrisisMMD: Multimodal X (formerly known as Twitter) datasets from natural disasters. In Proceedings of the International AAAI Conference on Web and Social Media, Palo Alto, California, USA. 2018; 12(1): 1-9. DOI: https://doi.org/10.1609/icwsm.v12i1.14983
Martín Y, Li Z, Cutter SL: Leveraging X (formerly known as Twitter) to gauge evacuation compliance: Spatiotemporal analysis of Hurricane Matthew. PLoS One. 2017; 12(7): e0181701. DOI: https://doi.org/10.1371/journal.pone.0181701
Yum S: Mining X (formerly known as Twitter) data to understand the human sentiment on Hurricane Florence. J Disaster Emerg Res. 2020; 3(2): 74-86. DOI: https://doi.org/10.18502/jder.4069
Yum S: The effects of Hurricane Dorian on spatial reactions and mobility. Nat Hazards. 2020; 105(3): 2481-2497. DOI: https://doi.org/10.1007/s11069-020-04408-8
Juran L, Trivedi J: Women, gender norms, and natural disasters in Bangladesh. Geogr Rev. 2015; 105(4): 601-611. DOI: https://doi.org/10.1111/j.1931-0846.2015.12089.x
Martín Y, Cutter SL, Li Z: Bridging X (formerly known as Twitter) and survey data for evacuation assessment of Hurricane Matthew and Hurricane Irma. Nat Hazards Rev. 2020; 21(2): 1-14. DOI: https://doi.org/10.1061/(ASCE)NH.1527-6996.0000354
West DM, Orr M: Race, gender, and communications in natural disasters. Policy Stud J. 2007; 35(4): 569-586. DOI: https://doi.org/10.1111/j.1541-0072.2007.00237.x
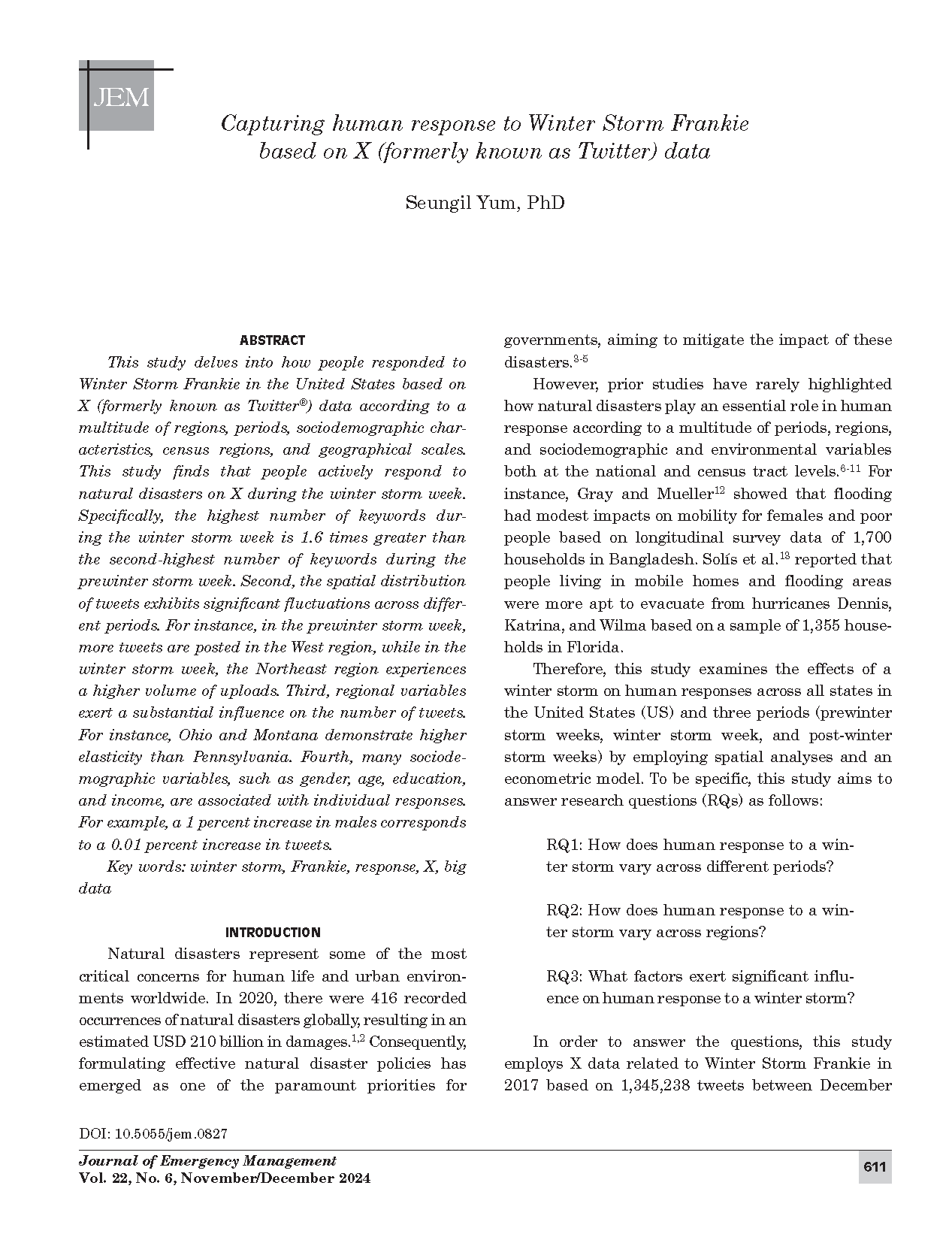
Published
How to Cite
Issue
Section
License
Copyright 2007-2025, Weston Medical Publishing, LLC and Journal of Emergency Management. All Rights Reserved.